5 reasons you should consider machine learning methods for assets price forecasting
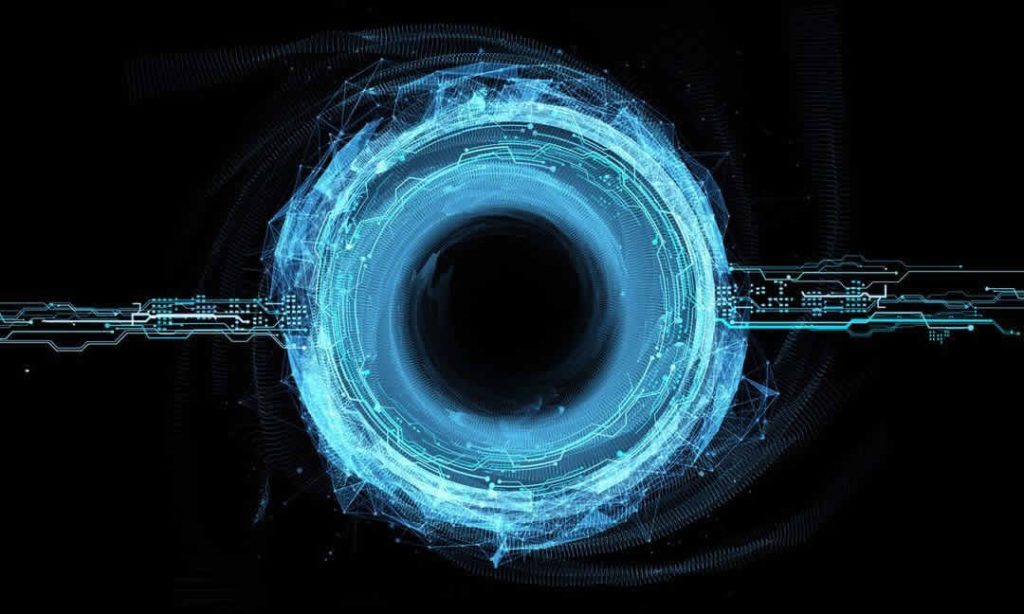
The financial industry has developed a variety of analytical tools such as fundamental analysis, technical analysis, and statistical analysis for asset prices forecasting. While these traditional analytical methods are useful, it’s no longer sufficient to compete and grow your business in this day and age.
How do you make sense from the ever-expanding volume of information? How do you separate alpha from noise to win in today’s capital market?
Using machine learning and deep learning to break through traditional limits
Machine learning models can be trained to dynamically generate predictions by taking in an enormous amount of historical and real-time information, and to learn, and adapt without pre-determined assumptions for a wide array of asset types such as equities, commodity, indices and more.
Kavout’s Kai forecast engine, for example, is a state-of-the-art AI service that analyzes, models and predicts equity movement for institutional customers. Kai can be customized according to asset classes, market cap, timeframes and even customers’ risk preferences. It provides forecast matrixes, including price range, probability of upward or downward movement and the corresponding confidence level.
Here are five reasons you should consider machine learning methods for price forecasting:
- Adaptability: Machine learning is self-learning, meaning that it keeps absorbing new information, and thus adapts to different market regimes promptly.
- Objectivity: Unlike statistical models, machine learning models don’t rely on human-derived assumptions. The human factor is only involved in building the mathematical framework and initial data gathering process.
- Reliability: Models can be assigned a confidence score, like what Kai forecast engine produces, to each forecast result for risk control. Investors can make decisions according to their own risk preferences.
- Scalability: Machines can scale a lot faster and cheaper allowing you to expand your coverage whether it’s stock, ETF, index, commodity or foreign exchange. Forecast can also be done for short-term, medium-term and long-term time horizon, spanning from three days to six months.
- Longevity: The performance of the prediction model can be tuned and algorithm enhanced as an ongoing effort to optimize forecast accuracy and output.
Again, using traditional analytical tools can yield good results. But money managers can’t expect to use tools that have been around for the past century and expect them to produce exceptional results. New challenges need to be solved by new solutions such as technological advancement in machine learning in identifying new trading opportunities.
Send us a Message
Contact us
Contact us today to learn more about Kavout's products or services.